It is a co-authored weblog from Professor Aleksandra Przegalińska and Denise Lee
As synthetic intelligence (AI) strikes from the hypothetical to the true world of sensible purposes, it’s turning into clear that larger is just not at all times higher.
Latest experiences in AI growth and deployment have make clear the facility of tailor-made, ‘proportional’ approaches. Whereas the pursuit of ever-larger fashions and extra highly effective methods has been a standard development, the AI neighborhood is more and more recognizing the worth of right-sized options. These extra targeted and environment friendly approaches are proving remarkably profitable in growing sustainable AI fashions that not solely scale back useful resource consumption but additionally result in higher outcomes.
By prioritizing proportionality, builders have the potential to create AI methods which are extra adaptable, cost-effective, and environmentally pleasant, with out sacrificing efficiency or functionality. This shift in perspective is driving innovation in ways in which align technological development with sustainability objectives, demonstrating that ‘smarter’ usually trumps ‘larger’ within the realm of AI growth. This realization is prompting a reevaluation of our elementary assumptions about AI progress – one which considers not simply the uncooked capabilities of AI methods but additionally their effectivity, scalability, and environmental impression.
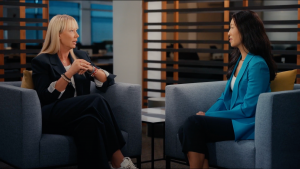
From our vantage factors in academia (Aleksandra) and enterprise (Denise), we’ve got noticed a vital query emerge that calls for appreciable reflection: How can we harness AI’s unbelievable potential in a sustainable approach? The reply lies in a precept that’s deceptively easy but maddeningly neglected: proportionality.
The computational assets required to coach and function generative AI fashions are substantial. To place this in perspective, think about the next information: Researchers estimated that coaching a single giant language mannequin can devour round 1,287 MWh of electrical energy and emit 552 tons of carbon dioxide equal.[1] That is corresponding to the vitality consumption of a median American family over 120 years.[2]
Researchers additionally estimate that by 2027, the electrical energy demand for AI may vary from 85 to 134 TWh yearly.[3] To contextualize this determine, it surpasses the yearly electrical energy consumption of nations just like the Netherlands (108.5 TWh in 2020) or Sweden (124.4 TWh in 2020).[4]
Whereas these figures are vital, it’s essential to think about them within the context of AI’s broader potential. AI methods, regardless of their vitality necessities, have the capability to drive efficiencies throughout numerous sectors of the know-how panorama and past.
As an example, AI-optimized cloud computing providers have proven the potential to cut back vitality consumption by as much as 30% in information facilities.[5] In software program growth, AI-powered code completion instruments can considerably scale back the time and computational assets wanted for programming duties, doubtlessly saving thousands and thousands of CPU hours yearly throughout the business.[6]
Nonetheless, hanging the stability between AI’s want for vitality and its potential for driving effectivity is strictly the place proportionality is available in. It’s about right-sizing our AI options. Utilizing a scalpel as a substitute of a chainsaw. Choosing a nimble electrical scooter when a gas-guzzling SUV is overkill.
We’re not suggesting we abandon cutting-edge AI analysis. Removed from it. However we will be smarter about how and once we deploy these highly effective instruments. In lots of circumstances, a smaller, specialised mannequin can do the job simply as nicely – and with a fraction of the environmental impression.[7] It’s actually about good enterprise. Effectivity. Sustainability.
Nevertheless, transferring to a proportional mindset will be difficult. It requires a stage of AI literacy that many organizations are nonetheless grappling with. It requires a sturdy interdisciplinary dialogue between technical specialists, enterprise strategists, and sustainability specialists. Such collaboration is important for growing and implementing actually clever and environment friendly AI methods.
These methods will prioritize intelligence in design, effectivity in execution, and sustainability in observe. The position of energy-efficient {hardware} and networking in information heart modernization can’t be overstated.
By leveraging state-of-the-art, power-optimized processors and high-efficiency networking gear, organizations can considerably scale back the vitality footprint of their AI workloads. Moreover, implementing complete vitality visibility methods offers invaluable insights into the emissions impression of AI operations. This data-driven method allows firms to make knowledgeable choices about useful resource allocation, determine areas for enchancment, and precisely measure the environmental impression of their AI initiatives. Consequently, organizations cannot solely scale back prices but additionally display tangible progress towards their sustainability objectives.
Paradoxically, essentially the most impactful and even handed software of AI would possibly usually be one which makes use of much less computational assets, thereby optimizing each efficiency and environmental concerns. By combining proportional AI growth with cutting-edge, energy-efficient infrastructure and strong vitality monitoring, we are able to create a extra sustainable and accountable AI ecosystem.
The options we create is not going to come from a single supply. As our collaboration has taught us, academia and enterprise have a lot to study from one another. AI that scales responsibly would be the product of many individuals working collectively on moral frameworks, integrating various views, and committing to transparency.
Let’s make AI work for us.
[1] Patterson, D., Gonzalez, J., Le, Q., Liang, C., Munguia, L.-M., Rothchild, D., So, D., Texier, M., & Dean, J. (2021). Carbon emissions and huge neural community coaching. arXiv.
[2] Mehta, S. (2024, July 4). How a lot vitality do llms devour? Unveiling the facility behind AI. Affiliation of Knowledge Scientists.
[3] de Vries, A. (2023). The rising vitality footprint of Synthetic Intelligence. Joule, 7(10), 2191–2194. doi:10.1016/j.joule.2023.09.004
[4] de Vries, A. (2023). The rising vitality footprint of Synthetic Intelligence. Joule, 7(10), 2191–2194. doi:10.1016/j.joule.2023.09.004
[5] Strubell, E., Ganesh, A., & McCallum, A. (2019). Power and coverage concerns for Deep Studying in NLP. 1 Proceedings of the 57th Annual Assembly of the Affiliation for Computational Linguistics. doi:10.18653/v1/p19-1355
[6] Strubell, E., Ganesh, A., & McCallum, A. (2019). Power and coverage concerns for Deep Studying in NLP. 1 Proceedings of the 57th Annual Assembly of the Affiliation for Computational Linguistics. doi:10.18653/v1/p19-1355
[7] CottGroup. (2024). Smaller and extra environment friendly synthetic intelligence fashions: Cottgroup.
Share: